AI in Risk Management
AI in Finance Nov 8, 2024 12:51:20 PM Mohamed Hassan 5 min read
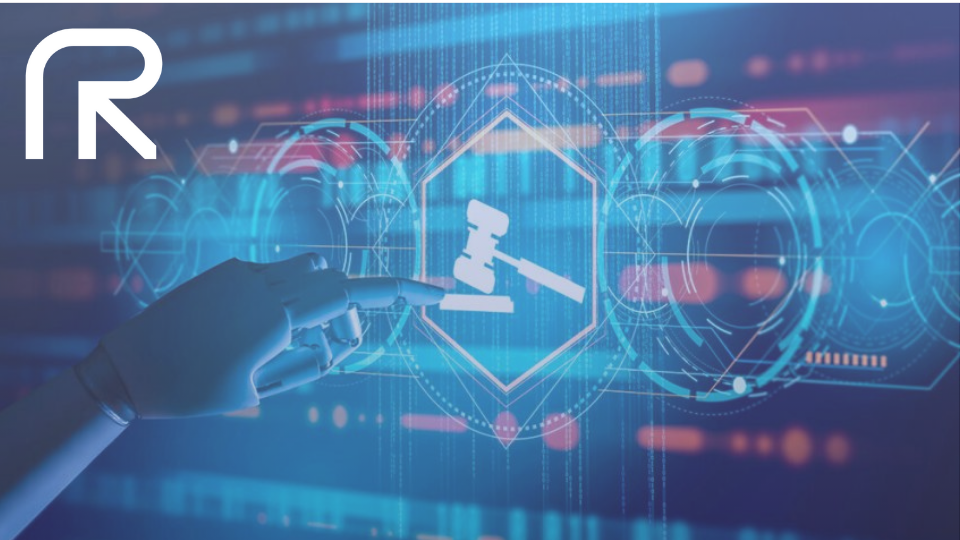
More than 80% of financial transactions in the USA and UK are now digital, opening up, not only, new opportunities, but also new challenges; as digitalization allows faster services and a wider reach, it also brings complexities that traditional risk assessment can’t fully handle.
Now financial institutions have to handle massive volumes of data in real time and react fast to shifting laws and threats. This where AI in finance plays a significant role.
Artificial Intelligence (AI) is stepping in to meet these demands by providing advanced insights, real-time monitoring, and predictive capabilities beyond traditional systems such as patterns spotting, and risks prediction.
Here are three key ways AI in risk assessment is making an impact.
Advanced Credit Scoring
Credit Scoring is one of the most common processes used by lenders to evaluate an individual's creditworthiness. It helps lenders decide whether to approve a loan or extend credit to an applicant.
For decades financial institutes relied on credit scoring methods that use static indicators like credit history, income, and existing debt to assess risk.
However, these conventional methods often fall short when evaluating applicants with limited financial history, or these models are vulnerable to fraud, as applicants with good financial history numbers might still engage in fraudulent behavior, making them risky borrowers despite seemingly strong scores.
AI-Powered Approach
A common process used by all lenders is credit scoring. credit scoring helps lenders decide whether to approve a loan or extend credit to an applicant.
Traditional credit scoring models use static indicators like credit history, income, and existing debt to assess risk.
Yet, static methods often fall short when evaluating applicants with limited financial history; lacking enough data to provide a full assessment.
They might also be vulnerable to fraud, since applicants with good financial history might still engage in fraudulent behavior, making them risky borrowers despite seemingly strong scores.
How It Works
1. Expanding Data Sources:
AI-powered credit scoring use a broader range of data, such as payment records, transaction histories, and even online behaviors, providing a more comprehensive view of an applicant's financial habits.2. Building Smarter Models:
Machine learning models—like gradient boosting algorithms and neural networks—analyze these diverse data sources to identify patterns in borrower behavior. This allows the model to make more accurate predictions about creditworthiness, especially for applicants who might not fit traditional criteria.
3. Ensuring Transparency and Compliance:
AI-driven credit models also focus on transparency. Tools like SHAP and LIME provide insights into why specific features (e.g., recent spending changes) affect an applicant's credit score, which helps meet regulatory requirements for explainability.A credit scoring system powered by AI allows for more accurate risk prediction. It makes it easy to analyze a broader range of factors, and can help financial institutions make more reliable credit decisions and better manage risk across a diverse applicant base.
Real-Time Fraud Detection
Wherever there’s money there’s fraud. That is why any financial institution must detect fraudulent transactions to prevent costly losses. Traditional rule-based systems are often rigid, producing high false positives and failing to keep pace with new fraud patterns.
AI-Powered Approach
Fraud detection when powered by AI leverage real-time anomaly detection, behavioral analysis, and ensemble models to accurately identify suspicious transactions and adapt dynamically to evolving fraud tactics.
How It Works
1. Real-Time Data Processing
AI-powered systems process transactions instantly using real-time data platforms like Apache Kafka. Each transaction is evaluated as it happens, with sliding time windows that focus on recent activity, enabling quick detection of unusual patterns.2. Advanced Anomaly Detection
Algorithms such as Isolation Forests and auto-encoders identify outliers by comparing transactions to typical patterns.Isolation Forests isolate anomalies, while autoencoders detect irregularities based on learned “normal” behaviors. By combining different models, AI can catch both known fraud signals and emerging fraud trends.
3. Behavioral Analysis
AI systems monitor user behaviors like login patterns and device changes, using RNNs to detect deviations that might indicate fraud. Graph-based models further map relationships between accounts and devices, helping to uncover suspicious connections and identify potential fraud rings.AI-powered fraud detection helps financial institutions reduce fraud losses and improve operational efficiency.
By accurately identifying and responding to suspicious activities in real time, these systems minimize false positives and enhance customer trust, creating a smoother experience for legitimate users.
Predictive Operational Risk Management
System failures, compliance breaches, human errors and other operational risks, can significantly impact financial stability.
Traditional risk management is often reactive and lacks the predictive capabilities needed to address these issues before they escalate.
AI-Powered Approach
AI flips the coin making risk management proactive rather than reactive by analyzing data from logs, employee activities, and external sources to identify risk signals early, enabling financial institutions to take preemptive action and reduce potential disruptions.
How It Works
1. Log Data Analysis with NLP and Sequence Models
AI analyzes logs, emails, and support tickets to identify risk-related terms and patterns. NLP tools pick up key phrases like "failure" or "outage," categorizing issues to reveal areas needing attention.
RNNs and Transformer models then process log data over time to detect sequences that often signal upcoming disruptions.
2. Predictive Maintenance for IT and Operational Assets
Machine learning models predict when systems or equipment might need maintenance, analyzing data like system usage, age, and error frequencies.Survival analysis models further estimate the timing of potential failures, helping to schedule maintenance before issues arise.
3. Automated Risk Scoring and Correlation of Risk Signals:
AI assigns a dynamic risk score to systems by continuously monitoring real-time data such as error logs and uptime.Bayesian networks map relationships between risk factors (like system performance and user activities), allowing AI to adjust risk predictions based on live conditions and detect new risks as they emerge.
With AI-powered predictive risk management, financial institutions can address risks proactively, preventing potential downtimes or regulatory issues.
This approach enhances operational resilience, reduces disruptions, and ensures compliance by focusing on early risk detection and timely interventions.
Risk assessment when powered by AI will exceed any levels of precision it reached before improving areas like credit scoring, real-time fraud detection, and predictive operational risk management leading to reduced losses, and rigid resilience against emerging threats.
Interested in learning how AI in risk management can power your institution’s capabilities?
Book a call with one of our AI experts to explore how custom AI solutions can address your specific challenges in risk assessment and beyond.
Mohamed Hassan
I’m a data analyst, writer, and consultant who's worked with everyone from scrappy startups to billion-dollar giants. On the side, I've built a 120K-strong community, Cats of Egypt, championing street cats, contributed to the growth of the Egyptian Professionals Network (EPN), and created a private haven for playful professionals. My writing gigs span over 100 organizations, including promoting Canada's AI mission for the Canadian Consulate in Miami and a major NFT conference in Asia. I’ve also helped shape the data analytics curriculum for one of USAID Egypt’s educational projects.