Data Analysts vs Data Scientists: Skills, Salaries, & Responsibilities
Apr 12, 2025 4:23:09 PM Mohamed Hassan 8 min read
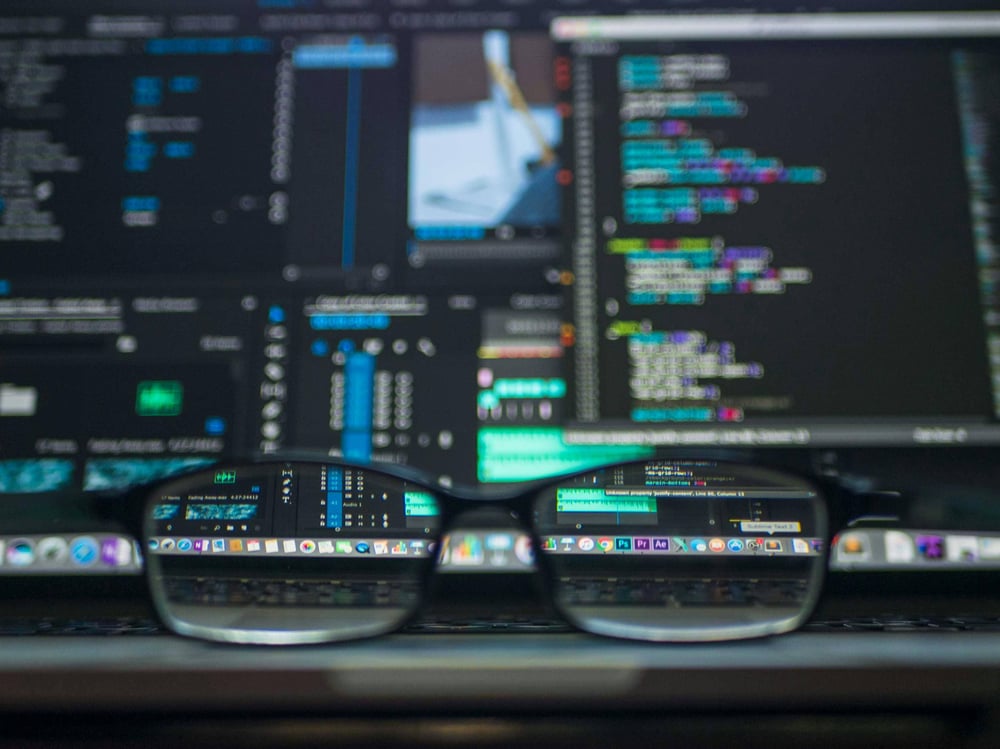
Data was once titled the king of growth, now AI is taking that title away however no AI can work without data, and to make the best out of data you need two roles: the data analyst and the data scientist.
At first glance, they might seem interchangeable, both deal with numbers, charts, and insights, right? But dig a little deeper, and you’ll find they’re as distinct as a painter and an architect.
Whether you’re plotting a career path, building a team, or just curious about the data universe, understanding what sets these roles apart is essential. So, let’s break it down: what exactly differentiates a data analyst from a data scientist, and why does it matter?
Overview of the Roles
Before diving into the nitty-gritty, let’s sketch a quick picture of each role. A data analyst is like a detective of the present, they take existing data, sift through it, and uncover stories that help businesses make sense of what’s happening. Think of them as the ones who turn raw numbers into actionable reports or eye-catching dashboards.
A data scientist, on the other hand, is more of a visionary. They don’t just look at what is, they predict what could be. Armed with advanced tools and techniques, they build models, run experiments, and craft solutions that anticipate the future. If data analysts tell you what happened last quarter, data scientists tell you what might happen next.
Both roles are vital, but their focus, tools, and approaches diverge in fascinating ways. Let’s explore those differences step by step.
Key Differences
Skills and Tools
The toolkit of a data analyst is practical and accessible. They’re wizards with spreadsheets (Excel is their trusty sidekick), fluent in SQL for querying databases, and skilled at whipping up visualizations in tools like Tableau or Power BI. Their statistical knowledge is solid but typically doesn’t venture beyond the basics—think averages, trends, and correlations.
Data scientists, by contrast, wield a more advanced arsenal. They’re coders at heart, often proficient in programming languages like Python or R. These languages let them manipulate massive datasets, build machine learning models, and automate complex processes. Their statistical toolbox is deeper, too, dipping into probability, regression analysis, and algorithms. While a data analyst might use a pre-built dashboard, a data scientist might code the dashboard from scratch—or create a predictive system to feed it.
Responsibilities
Day-to-day, a data analyst’s job revolves around clarity and answers. They clean messy data (a process called data wrangling), spot patterns, and translate findings into reports or presentations. Imagine a retail chain asking, “Why did sales dip in July?” A data analyst would dig into the numbers, chart the trends, and deliver a clear explanation—perhaps tied to weather or a marketing misstep.
Data scientists tackle bigger, fuzzier questions. Their work leans toward experimentation and innovation. That same retail chain might ask, “How can we boost sales next July?” A data scientist would analyze past data, build a predictive model (say, forecasting demand), and maybe even recommend a pricing strategy based on machine learning insights. They’re less about reporting and more about creating—think algorithms over pie charts.
Education and Background
You don’t need a PhD to be a data analyst, though many have bachelor’s degrees in fields like business, economics, or statistics. Their skills can often be honed through online courses or on-the-job experience, making the role more accessible to newcomers. Certifications in tools like SQL or Tableau can seal the deal.
Data scientists, however, often come with heavier academic baggage. Many hold master’s degrees or PhDs in computer science, math, or related disciplines. Why? Their work demands a grasp of complex concepts—think linear algebra for machine learning or calculus for optimization. That said, self-taught data scientists do exist, piecing together expertise through coding bootcamps and real-world projects. Still, the bar is higher, and the learning curve steeper.
Problem-Solving Approach
Here’s where the philosophical divide shines. Data analysts are masters of the descriptive. They answer “what happened?” and “why did it happen?” with historical data. Their work is grounded in the concrete—sales figures, customer behavior, operational metrics.
Data scientists, meanwhile, lean into the predictive and prescriptive. They ask, “What will happen?” and “What should we do about it?” Their answers come from models that forecast outcomes or simulations that test scenarios. A data analyst might tell you website traffic spiked on Black Friday; a data scientist might predict next year’s spike and suggest how to optimize server capacity.
Aspect |
Data Analyst |
Data Scientist |
Skills |
Excel, SQL, Tableau, basic stats |
Python, R, machine learning, advanced stats |
Responsibilities |
Reports, dashboards, data cleaning |
Predictive models, algorithms, experiments |
Education |
Bachelor’s, certifications |
Master’s/PhD, specialized training |
Approach |
Descriptive (what/why) |
Predictive/prescriptive (what’s next) |
Real-World Applications
To bring this to life, let’s look at how these roles play out in the wild. Picture a streaming service like Netflix. A data analyst might pore over viewing data to report which shows topped the charts last month. They’d create a dashboard showing trends, maybe documentaries surged in winter, and share it with the marketing team to refine campaigns.
Now, a data scientist at Netflix? They’re the ones powering the recommendation engine. They’d take that same viewing data, mix in user preferences, and train a machine learning model to suggest your next binge-watch. Their work doesn’t just explain the past, it shapes your future viewing experience.
Or healthcare. A data analyst might track patient wait times in a hospital, identifying bottlenecks and suggesting staffing tweaks. A data scientist might analyze patient records to predict disease outbreaks, helping the hospital prepare months in advance. Same data, different horizons.
Salary Ranges: Data Analyst vs. Data Scientist
- United States: Data analysts earn $65,000–$95,000 annually, with entry-level roles starting at $60,000 and senior positions exceeding $100,000 in tech hubs like San Francisco.
Data scientists average $100,000–$150,000, with juniors at $85,000 and seniors in specialized roles hitting $200,000+ at top firms. - Europe: Data analysts make €40,000–€65,000 ($43,000–$70,000 USD), ranging from €30,000 in Spain to €80,000–€100,000 in Switzerland.
Data scientists earn €50,000–€90,000 ($54,000–$97,000), with highs of €100,000–€140,000 in Switzerland and lows of €40,000 in Southern Europe. - Global: Globally, data analysts average $60,000–$80,000 USD, with India at $10,000–$30,000 and Japan at $40,000–$70,000.
Data scientists range $75,000–$100,000, from $15,000–$60,000 in India to $90,000–$130,000 in Canada or Australia.
The salary gap reflects data scientists’ advanced skills in coding and predictive modeling versus analysts’ focus on accessible tools and descriptive insights. US salaries lead due to tech demand, while Europe and global markets vary by economic conditions.
Overlap Between the Roles
Despite their differences, data analysts and data scientists aren’t entirely separate species. Both need a knack for numbers and a curiosity about what data can reveal. They share foundational skills, data cleaning, basic analysis, and a love for problem-solving. In smaller companies, the lines blur even more. A data analyst might dabble in simple predictive models, while a data scientist might whip up a quick report when deadlines loom.
The overlap shrinks as organizations grow, though. Big firms with dedicated data teams carve out distinct roles: analysts feed insights to scientists, who then build the heavy-duty solutions. Think of it as a relay race, analysts run the first leg, scientists the second.
Which Role Is Right for You?
If you’re reading this with a career in mind, here’s a quick gut check. Love structure, detail, and turning chaos into order? Data analysis might be your sweet spot. You’ll thrive if you enjoy solving puzzles with clear boundaries and presenting answers in a polished package.
Drawn to the unknown, happy to experiment and fail, and eager to code your way through ambiguity? Data science could be your calling. It’s a role for tinkerers and big-picture thinkers who don’t mind getting their hands dirty with complex tools.
Of course, there’s no one-size-fits-all. Some start as analysts and evolve into scientists as they pick up skills. Others stick with analysis, mastering its craft. It’s less about “better” and more about fit.
So, what’s the takeaway?
Data analysts and data scientists both wield the power of data, but they aim it in different directions. Analysts shine a light on the present, delivering clarity and answers from what’s already there. Scientists gaze into the future, crafting tools and insights to shape what’s coming. One’s not superior to the other—they’re complementary, like two sides of a coin.
For businesses, this means hiring the right role for the right problem. For individuals, it’s about aligning your strengths with your goals. Whether you’re drawn to the precision of analysis or the creativity of science, the data world has a place for you. So, which path will you explore?
Mohamed Hassan
I’m a data analyst, writer, and consultant who's worked with everyone from scrappy startups to billion-dollar giants. On the side, I've built a 120K-strong community, Cats of Egypt, championing street cats, contributed to the growth of the Egyptian Professionals Network (EPN), and created a private haven for playful professionals. My writing gigs span over 100 organizations, including promoting Canada's AI mission for the Canadian Consulate in Miami and a major NFT conference in Asia. I’ve also helped shape the data analytics curriculum for one of USAID Egypt’s educational projects.